Contents
Numerai: Decentralized Stock Market Modeling via Erasure
An old standby, the stock prediction model reaches new heights with Numerai‘s transformative blend of AI and blockchain, complete with its NMR tokenized incentive reward system.
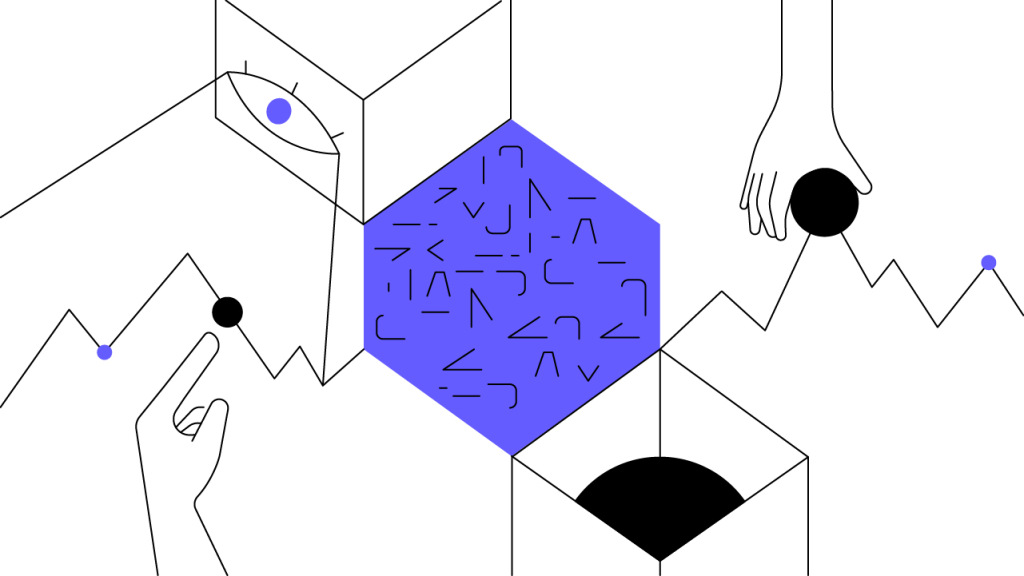
Summary
Established in 2015, Numerai is a blockchain-powered and AI-enabled hedge fund focused on improving stock market inefficiencies by aggregating high-quality crowdsourced stock market prediction models. The company transforms and normalizes financial data into machine learning (ML) problems that can be tackled by a global community of data scientists, and releases these problems via weekly competitions that incentivize high-quality contributions. Contributors within Numerai’s prediction ecosystem are rewarded in Numeraire (NMR), Numerai’s native cryptocurrency, as an incentive for submitting original and accurate market predictions. Numerai ensembles the best submissions into a meta model which is used to trade the stock market. Numerai’s industry-leading prediction tournament provides encrypted data sets, which anyone in the world can download for free and model. When participating in the tournament, participants retain their algorithms and submit their predictions only. The company has also recently launched a new prediction tournament which allows contributors to submit market signals using their own data sources.
A Look At Traditional Hedge Funds
Increased market volatility and bearish economic expectations traditionally drive investor interest in hedge funds — which aim to achieve absolute returns regardless of macroeconomic conditions, and collectively account for trillions of dollars’ worth of assets under management.
However, hedge funds are also notoriously opaque (given their interest in maintaining a competitive edge), and are therefore financially disincentivized from sharing their respective data sets and overall approaches with the rest of the market. Hedge funds possess limited means of determining the originality of the market signals on which they base their predictions or whether they are making predictions based on a subset of variables that a competitor has already modeled out. In other words, it’s hard for hedge funds to tell whether they’re repeating work that dozens or even hundreds of competitors have already mastered, but many hedge funds would rather risk wasting time and money than risk giving away a potential competitive edge. As a result, most hedge funds are unable to consistently beat the market over the long run, given that the sector is rife with hidden redundancies and inefficient investment allocations.
Introducing Numerai’s Open Hedge Fund Concept
Numerai’s novel approach to stock market forecasting aims to disrupt the traditional hedge fund industry paradigm. Numerai opens up the process of market modeling to a broader range of anonymous participants, and incorporates these participants’ best-performing algorithmic contributions, which are machine learning models, into Numerai’s own meta model.
Given that there is no universal, timeless approach that can consistently beat the markets, the trading algorithms underpinning a predictive model need to be frequently updated as new information is made available. Furthermore, the benefits of a meta model (composed of multiple independently generated models) extends beyond potential increases in returns. For example, a meta model can support the optimal functioning of a fund of funds, which is based on multiple independently generated models, and contains many more independent bets than a portfolio built around a single model. The intrinsic diversification that this approach enables can lead to lower error rates in , promote reduced portfolio risk, and ultimately increase a fund’s ability to take on more leverage.
Encouraging the consistent creation and improvement of multiple high-quality and independently generated models is vital to the proper functioning of the Numerai platform. As a result, the success of its approach relies not only on Numerai’s effective conversion of financial data sets into encrypted yet solvable machine learning problems, but also on the company’s ability to incentivize a global community of data scientists to solve these machine learning problems over the long run.
Numerai’s Encrypted Stock Prediction Tournament
In order to incentivize participation in their model, Numerai created the world’s first encrypted data science tournament for stock market prediction models back in December 2015. This tournament was the company’s first intelligence crowdfunding initiative, and has grown to become the largest stock market data science tournament in the world.
Numerai’s competition is divided into weekly rounds, with each round lasting for one month. Each week, Numerai releases a new set of encrypted financial data, which anyone can download and build a predictive model around. The data is provided in CSV format, and consists of a set of training data (containing rows of features and their target values), as well as a set of tournament data which participants can use to run their trained models against to generate their predictions.
Furthermore, the competition’s data sets are encrypted in a way that preserves the integrity and structure of the underlying data while obfuscating the true nature of the information itself. As a result, the data sets are malleable, and can be used within a machine learning prediction context without revealing the specifics of what is being solved. Upon submission, Numerai assesses a participant’s predictions via in-house validation, tests, and live sets known only to Numerai — and rewards the winners based on the results of these procedures. In this way, the weekly market modeling problems Numerai issues are reduced to pure, context-free mathematical exercises. While tournament participants are therefore unable to gain insights into how exactly their predictions are contributing to Numerai’s meta model, Numerai cannot reverse-engineer participants’ models either, thereby ensuring a balanced, mutually beneficial dynamic. All parties involved can maintain the integrity of their respective proprietary models while simultaneously minimizing any unnecessarily repetitive work.
In order to attract capable contestants to this weekly tournament, Numerai offers compelling financial incentives for participants who submit the most original and accurate market signals, and has already paid out tens of millions of dollars in cryptocurrency rewards to its weekly tournament winners. While participants do not invest in or generate any income from the hedge fund directly, they receive a portion of the prize earnings relative to the predictive accuracy and originality of their contributions. In this context, originality is a measure of whether a set of predictions is uncorrelated with predictive market signals that have already been submitted. The goal is to prioritize originality as a means of limiting duplicate submissions, while also encouraging new models and innovative approaches. Numerai has fostered a network of more than 30,000 active participants, and more than 1,200 staked models are incorporated into Numerai’s meta model every week.
The Evolution of NMR’s Utility: Tournaments to Erasure Protocol
While Numerai’s tournament rewards were originally paid out first via Paypal and later in bitcoin (BTC), in 2017 Numerai launched its own native ERC-20 token, , which participating data scientists now use to stake in tournaments. In order to receive tournament winnings, participants must stake their submissions using NMR tokens. Winning selections receive a commensurate portion of the prize money and participants can earn up to 25% of their stake every week. Conversely, if participants fail to beat the benchmark, up to 25% of their staked NMR is burned — permanently reducing the total supply of NMR tokens.
While NMR was initially created to coordinate and align the incentives of a global network of data scientists in Numerai’s tournament, it is expanding to address uses far beyond the Numerai tournament. In 2018, Numerai set out to expand the potential use cases of NMR with the launch of the Erasure Protocol. Erasure is an open, all-encompassing staked information ecosystem that embodies Numerai’s end goal of building the largest ensemble of machine learning models in the world.
The launch of Erasure Protocol and its associated dApps initiated NMR’s transition away from a centralized token exclusively incentivizing Numerai data scientists and toward a decentralized blockchain protocol that anyone can build upon and use. Additionally, around the same time as the Erasure Protocol launch, Numerai permanently “halved” (roughly speaking) the supply of NMR tokens from 21 million to 11 million and ended the company’s ability to mint new NMR tokens. For a better understanding of Numerai’s ongoing efforts to advance NMR and Erasure, read this article about .
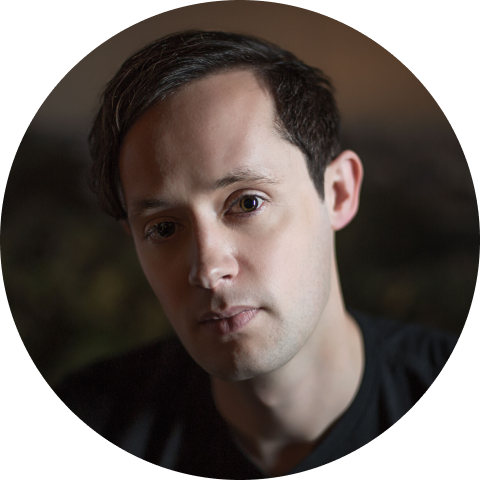
Author
Founder & CEO, Numerai
Richard Craib is the founder and CEO of Numerai, based in San Francisco. He graduated from Cornell University with a BA in Mathematics in 2012 with a focus in abstract algebra. He has also taken classes at UC Berkeley, Stanford, and the University of Cape Town. Before starting Numerai, Craib was a quant at an asset management firm with $15 billion in assets under management in Cape Town, South Africa, where he built a global equity long-only fund powered by machine learning.
Follow me on:
Is this article helpful?